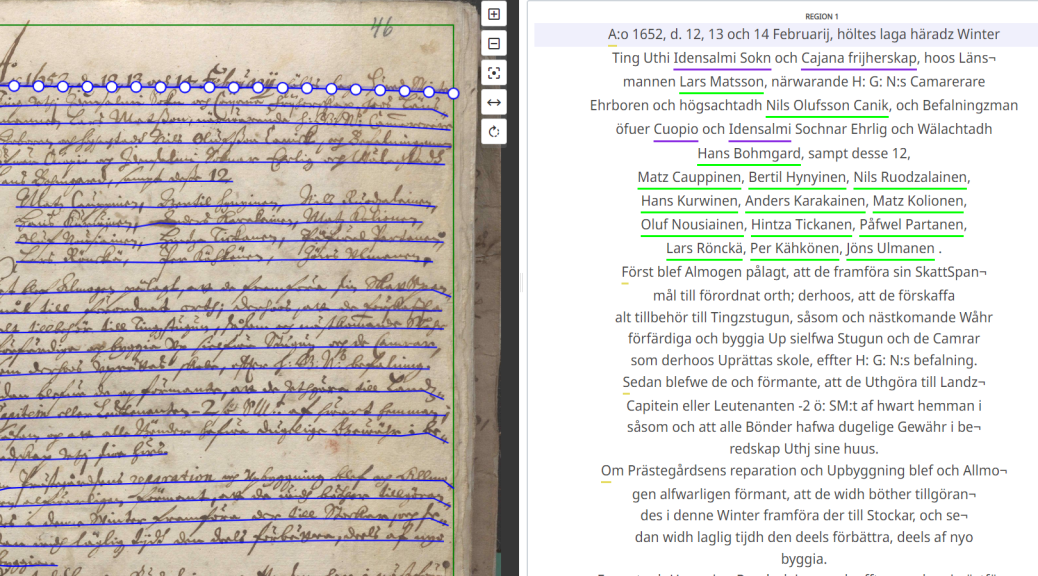
New 17th-Century Swedish-Recognizing HTR Model Published
Handwritten Text Recognition (HTR) is an AI-based technology that allows historical handwritten sources to be converted into machine-readable and digital format. This technology is fairly new, with most development occurring during the EU-funded READ (Recognition and Enrichment of Archival Documents) project from 2016 to 2019. Unlike Optical Character Recognition (OCR) for printed text, HTR requires the user to first teach the machine to read old handwriting before it can make interpretations. The work is done through a program called Transkribus, which generally does not read all handwriting; separate models must be created for different handwriting types. Even though models can be shared with the public, one of the biggest challenges is getting users to work together and share their models with each other.
It gives me great pleasure to share that I have now published my own HTR model that is adept at recognizing 17th-century Swedish, which is now available for use in the Transkribus program. This is a significant contribution to early modern historians, as the model is specifically designed to read the so-called ’New Gothic’ script of the 17th century, also known as the ’German script’. The model, named ’Swedish 17th Century (Savo, Eastern Finland)’, can be accessed by all interested researchers in the public models section of the program. At the moment, Transkribus provides 500 free credits to new users of the program, in addition to which, researchers working on their master’s or doctoral thesis can apply for free processing through the developers.
In HTR technology, the model’s ability to understand handwriting is dependent upon the training it has undergone. My model includes training data from various document collections held in the National Archives of both Finland (Kansallisarkisto) and Sweden (Riksarkivet). The majority of the training material consists of court records. As a whole, the model incorporates:
• District court (Finnish: kihlakunnanoikeus, Swedish: häradsrätten) records related to the Iisalmi parish from 1639–1699 (jurisdictions of Savolax 1639–1650, Kajana friherreskap 1651–1680, Lilla Savolax 1681–1699)
• Mentions of Iisalmi parish residents in the lagmansrätt (Finnish: laamanninoikeus) records from 1643–1699 (Legal district of Karjala, Karelska lagsagan)
• Letters sent to Count Per Brahe by local officials, clergy, and townspeople (Skokolstersamlingen, Rydboholmssamlingen)
• The complaints of the common people (Finnish: rahvaanvalitukset, Swedish: allmogens besvär) from the jurisdiction of Lilla-Savolax (Finnish: Pien-Savo)
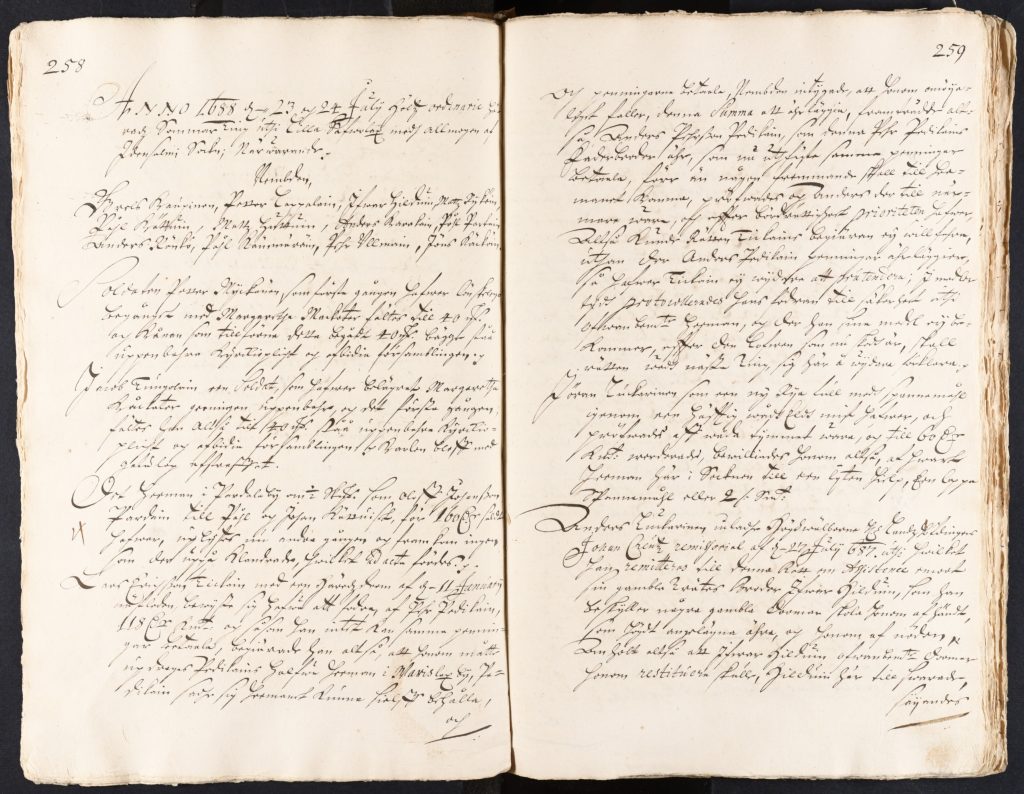
How and Why Was the Model Created?
The idea of developing my own HTR model was born when I was working on the READ project at the National Archives in 2019, where automatic recognition of handwritten text was being used on 19th century court records. I joined the project at a point when significant results were already being achieved with the launch of the HTR+ engine. I was naturally impressed with how well it could read 19th-century handwriting, and I wanted to test it on my own material, although I knew that the 17th-century handwriting would likely be more challenging for the AI to interpret and the quality of the existing digitized images was during that time substantially poorer.
Simultaneously, as I began working at the National Archives, I also embarked on postgraduate studies at the University of Helsinki. In my dissertation, I’m examining the progression of state formation in the parish of Iisalmi from 1639–1699. My primary source material is the 17th-century district court records. I had already used court records in my master’s thesis, during which I faced challenges interpreting the handwriting. Given the gap between my thesis and the start of my doctoral work, I became concerned about how I would interpret my source material at the required level. For these reasons, I thought that technology could aid in understanding old handwriting and perhaps even facilitate the task. As everyone who uses digital research methods knows, one can hardly speak of ”saving time”. However, I do not regret the hours spent with Transkribus, as painstaking transcription of each word forced me to learn 17th-century handwriting. I can recommend the use of Transkribus to other researchers for learning to read any old handwriting.
Developing my now-published HTR model required a significant personal commitment. While working at the National Archives, I started to create the model as an ”evening job” and could devote myself to it full-time in the fall of 2020 when I received funding to become a full-time doctoral researcher. The majority of the material in the model was created between 2020–2021, and I have not significantly updated it since my main work with the source material has ended. Originally, I had thought to publish the model only in conjunction with my dissertation, but I decided to publish it now when the final funding for my dissertation was secured from the SLS Foundation.
How Has the Model Developed and What Are Its Limitations?
I started developing the model from 1680s court records, as the handwriting of the scribe during this period was familiar to me. At that time, there were no other models available (currently, the public models also include a model specialized for the 17th-century court records of Jämtland province, Sweden), necessitating manual transcription of the records. The first model was based on 12 double-pages of court records and yielded a promising Character Error Rate (CER) of 13 %, meaning it could correctly recognize 87 out of 100 characters. Initial test were encouraging, especially when the model was tested on material from the same scribe. Despite its shortcomings, it was quicker to correct the model’s errors than to transcribe everything manually. However, the model’s performance dropped noticeably when confronted with another scribe’s slightly different handwriting style.
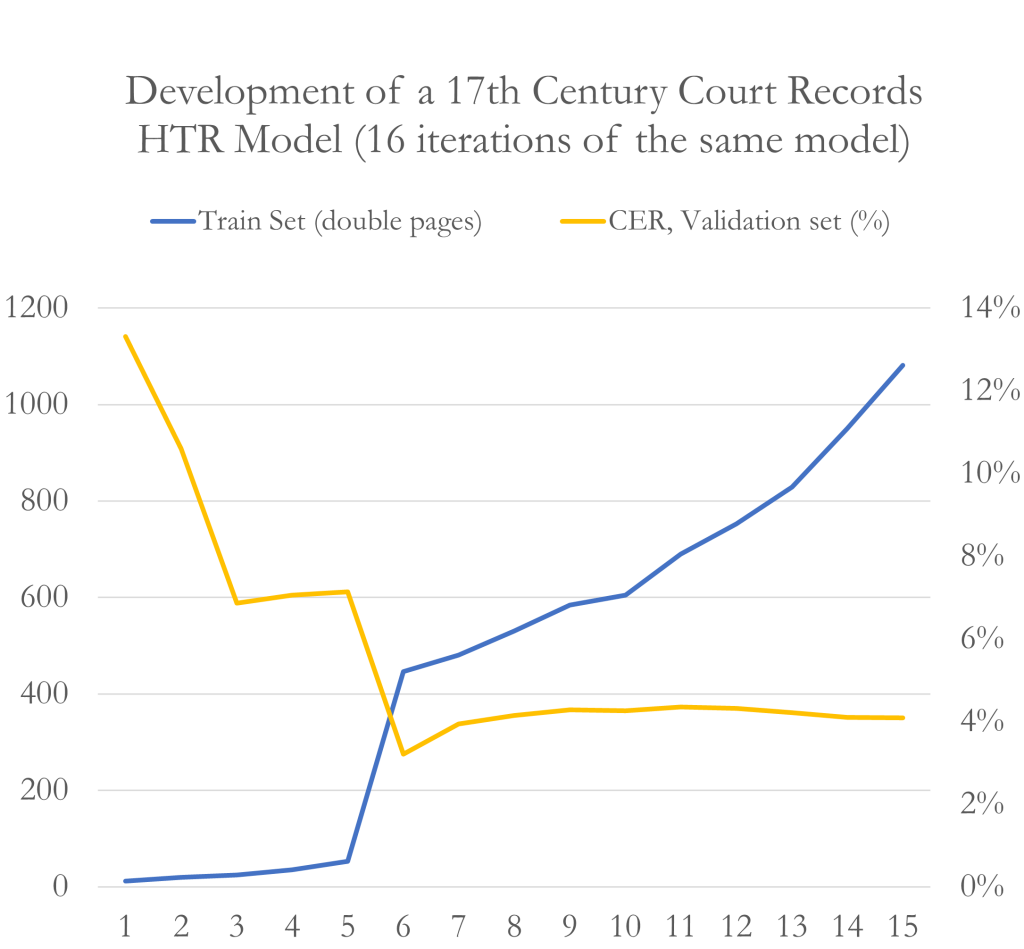
Today, my model demonstrates a competent ability to read random 17th-century Swedish ’New Gothic’ script. The CER for the validation set stands at 3.8 %, which is concerned to be a good result. However, the more I have worked with Transkribus, the less I believe that CER alone is a reliable indicator of a model’s quality. This is because the validation set would need to be more extensive and include unfamiliar handwriting styles. Only through testing can one truly determine how well the model is able to interpret different handwriting styles. From a model development perspective, I would appreciate it if the individuals who have tested the model could also share their experiences regarding the types of data it performs well with, as well as the types of data that present challenges.
Given my geographical focus, which is primarily the parish of Iisalmi with some inclusions from other areas, the model’s ability to read proper names (both personal and place names) is somewhat limited. HTR models that utilize machine learning risk overfitting if the training material does not adequately represent the material under study. If the primary goal of my doctoral project had been to create a model that could read 17th-century handwriting as effectively as possible, I would have selected material from different scribes and geographical areas. Now the artificial intelligence has mainly been taught a very limited number of different surnames and place names. Because these occur so frequently in the training material, it is very likely that a researcher whose material reads Leppänen and Perttunen will get recognition results where these names have been interpreted as Lappalainen and Partanen, as these are the most common surnames in Upper Savo (Ylä-Savo).
Additionally, the distribution of training material over time is uneven, since there is more training material from the end of the 17th century. This bias is due to the increased frequency and duration of court sessions during this period, with the length of court records surging dramatically from a single double-page record in 1639 to as many as 38 double-pages in 1693. Similar biases are present in other material, mostly originating from the end of the 17th century. For instance, more complaints from the common people have survived from the latter half of the century. Conversely, the Count Per Brahe letter collection from 1650–1680 offers a slight counterbalance.
When preparing training material, the researcher should be very consistent in how they transcribe historical sources. For machine learning, it would be best to operate systematically and in the same way in every situation. This is somewhat more achievable for individual researchers, while group-based researchers must communicate extensively about transcription rules. However, it is crucial even for individual researchers to document their process, a step unfortunately overlooked in this model’s development. This has likely led to some inconsistencies in the model that would require work to correct. Some of them relate to my personal learning process, I myself did not know the material well enough at first to define perfect practices, although fortunately working in the National Archives project gave me some examples to follow. One example of inconsistency is marking the letter ”k” in many places with a capital and sometimes with a lowercase, as I could not perceive the difference between a capital and a lowercase ”k”.
Similar mistakes can be also found. For several scribes, certain letters also look the same, and I probably did not always know to systematically decide whether to mark a letter as ”a” or ”e”. The genitives of the Swedish language, which are marked as underlines, are sometimes ”s”, sometimes ”z”, and sometimes left unmarked. In general, I have favored efficiency over precision in transcribing, so there would probably be a lot to correct in the training material, especially now that I can read the handwriting better.
In general, I have followed the principle that the transcription should remain faithful to the original source. Consequently, I have not expanded abbreviations. For commonly occurring abbreviations, I have maintained a specific way of noting them down. For instance, the currency thaler (Swedish: daler) is transcribed as either ”D” or ”D:r”, and the mark as either ”m” or ”m:r”, depending on the scribe. My goal has been to convey the scribe’s intent, while respecting the variations in spelling for the same words. In cases of uncertainty, I have used the unclear tag provided in Transkribus, and these lines were excluded from the training material when creating the model.
Although I cannot say with certainty, I speculate that the diversity in image quality of the training material has influenced my model’s recognition capabilities. The model’s development primarily took place before the National Archives of Finland initiated the redigitization of the 17th-century court records. Consequently, I had to rely on digitizations made from microfilms created in the 1950s. The quality of these black-and-white images ranged from excellent to extremely poor, with many images requiring processing with photo editing software before being input into the program. I also photographed parts of the district court records in the National Archives of Finland myself, with special permission. The material sourced from the Swedish Riksarkivet also varied in quality. Some pages were captured with a mobile phone camera, others digitized from microfilm, and yet others were high-quality digitizations from the original source. As a result, the model’s performance might improve if the task was carried out on a more uniform and higher-quality set of images.
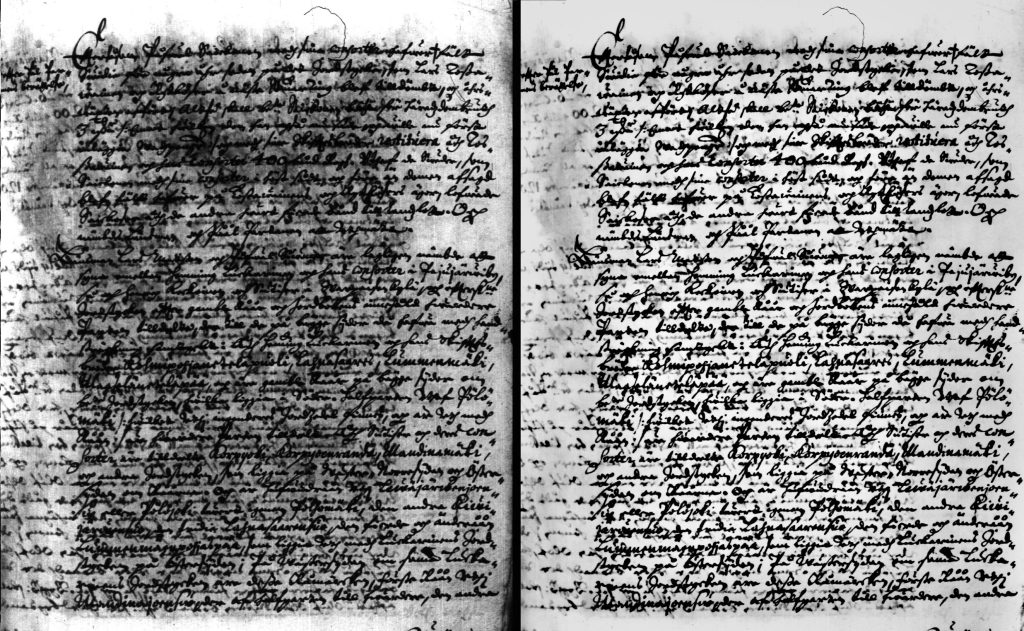
What are the Advantages of the HTR Model and the Future Prospects?
The HTR processing and model creation constituted a relatively small segment of my ongoing doctoral research. After the model’s construction, I have established a research database from the material, wherein each entry represents an individual court case. The creation of this research database might have been possible without transcriptions, but it might have remained unfinished. In the database, I include, for instance, legal history data of all individuals cited in court, which, to my knowledge, is a fairly unique approach to 17th-century court records.
During my master’s studies, I did not participate in any specific methodological courses, and Transkribus served as a springboard for my exploration of other digital methods applicable to 17th-century court records. Having sources in a digital and structured format enables the use of different methodologies. Besides traditional descriptive tables and charts, I have, for example, incorporated network analysis into my data. I have also developed an interest in mapping data using the QGIS program and experimenting with spatial analysis of land disputes reflected in court records.
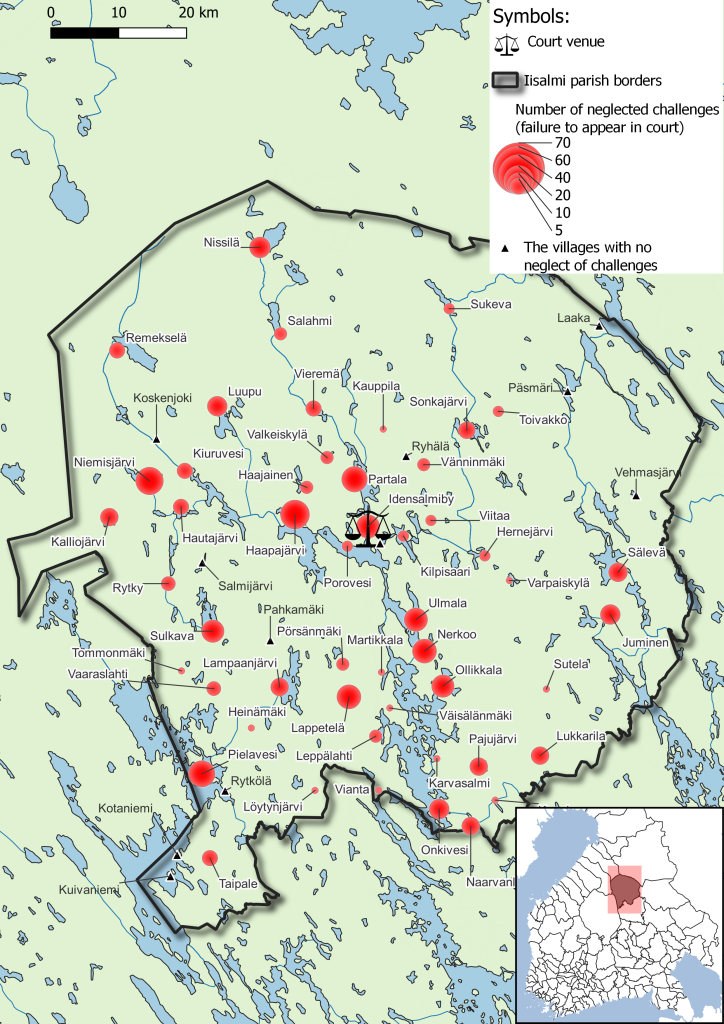
It is worth emphasizing that HTR introduces many new opportunities for a researcher primarily focused on qualitative research. Although I have reviewed the court records twice, identifying individual cases and case histories pertinent to my research themes without the database and transcriptions would have been a challenge.
After completing work on Iisalmi’s court records, I have sporadically transcribed new teaching material. Typically, I use the model to speed up information retrieval, thus I run my model and use the transcription for data extraction rather than correcting mistakes made by the model, which does not generate new teaching material. Nevertheless, my future intentions still include model development. At this point, it also seems logical to evolve the model in collaboration with other researchers.
Being a board member of the newly established association, ‘Network for Early Modern (ca 16th–17th century) Research in Finland’ (Uuden ajan alun tutkimuksen verkosto ry), I share a mutual interest with other board members in developing HTR models for earlier periods. Specifically, our goal is to develop a better model focused on the recognition of 17th-century Swedish language. We have initiated preliminary cooperation with the National Archives of Finland towards this end, and releasing my model publicly is the first step in this collaboration. Our objective is to create a temporally and geographically diverse model capable of interpreting 17th-century handwriting from a variety of source material as accurately as possible. One of the substantial challenges of HTR processing is generating training material. If you are interested in jointly developing an improved model, sharing your own data, or participating in source transcription, please contact:
ville-pekka.kaariainen@helsinki.fi or the association’s email address directly at toimikunta@uudenajanalku.fi.
Acknowledgements
Firstly, my gratitude goes to all the developers and maintainers of Transkribus. I have been operating during a period when the program was still free, and I also received complimentary credits from the program developers as part of the Scholarship Programme to process my material. I extend my thanks to the National Archives of Finland, especially to Maria Kallio who led the READ project, as well as other people who worked in the team. Secondly, I express my gratitude to my supervisor Anu Lahtinen, who introduced me to the world of old handwriting. Lastly, I would like to acknowledge all the funders of my doctoral dissertation, including the OLVI Foundation, the Finnish Cultural Foundation, the Emil Aaltonen Foundation, and the Svenska litteratursällskapet i Finland (SLS Foundation).
Ville-Pekka Kääriäinen
The author is an MA and currently a PhD researcher at the University of Helsinki. The doctoral research focuses on the progress of state formation in the parish of Iisalmi (Upper Savo) during the years 1639–1699.
Cover Image:
Screenshot from the Transkribus Lite. Winter court of Iisalmi parish 1652. National Archives, jurisdiction of Kajana Friherreskap. Person names are tagged in green and place names in violet. Errors in the transcription have been corrected.
Links:
The description details of the model in the Transkript’s public collection of models. Retrieved June 6, 2023, from here
Sources:
Kääriäinen, Ville-Pekka (2021). Käsin kirjoitetun tekstin automaattinen tunnistus (Automatic Handwritten Text Recognition) in Vanhojen käsialojen lukuopas (Old Handwriting Manual), edited by Petteri Impola, Pirita Frigren, Petri Karonen, Matti Roitto, Antti Räihä, & Jari Eilola. Helsinki: Gaudeamus.